AACR Virtual Annual Meeting II: Bioinformatics and Artificial Intelligence Fuel New Cancer Discoveries and Ideas
“Progress in science depends on new techniques, new discoveries, and new ideas— probably in that order,” said the late Nobel Laureate and molecular biologist Sydney Brenner, MBBCh, DPhil, FAACR.
In an introduction to the Bioinformatics and Artificial Intelligence minisymposium at the AACR Virtual Annual Meeting II, Jonathan Dry, MSc, session moderator and vice president of scientific discovery at Tempus Labs, revisited this quote from Brenner. Dry explained that recent breakthroughs in new techniques have allowed researchers to produce and utilize large cancer datasets and have enabled new discoveries and ideas. The advent of artificial intelligence and machine learning have supplied computational biologists with innovative tools to better analyze these data, providing new clues to cancer biology, diagnosis, and treatment.
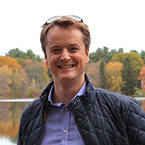
Some of the latest advances were discussed during the session, which featured researchers who used bioinformatics and/or artificial intelligence to better understand cancer biology, to identify therapeutic targets, or to provide platforms that aid researchers or clinicians in their work.
Understanding Cancer Biology
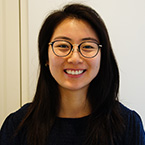
Two of the studies presented during the session used single-cell technologies to further the field’s understanding of cancer biology and antitumor immune responses. The session began with a talk from Xingyi Shi, a PhD candidate at Boston University, who performed single-cell profiling on patient lung samples to identify gene expression differences between lung squamous cell carcinomas and lung adenocarcinomas. The analysis identified 247 genes that were upregulated in lung adenocarcinomas as compared with lung squamous cell carcinomas. These genes were associated with ionocytes and ciliated cells and were enriched for pathways related to cilium morphogenesis, cilium organization, and regulation of p53 signaling. Shi also found 204 genes upregulated in lung squamous cell carcinomas, which were associated with secretory cells and were enriched for pathways related to TNFα and NFκB signaling, keratinization, and epithelial-mesenchymal transition. The gene expression differences identified in this study could contribute to an improved understanding and treatment of these lung cancer subtypes.
In another presentation, Edgar Kozlova, PhD, a postdoctoral researcher at the Icahn School of Medicine at Mount Sinai, described the use of single-cell transcriptomics to compare transcription profiles between tumor antigen-responsive (immunized) and non-responsive (tolerized) CD4+ T-cell subsets after vaccine immunotherapy. While the expression of genes within a tolerized gene set differed between the T-cell subsets, the researchers found that expression of T-cell exhaustion genes did not significantly differ between immunized and tolerized CD4+ T cells. Kozlova and colleagues found that tolerized T cells exhibited high clonal diversity and poor antigen stimulation. Consistent with the gene expression differences between the subsets, the researchers also identified differences in transcription factor activity between immunized and tolerized T cells. This study revealed distinct transcription profiles between immunized and tolerized CD4+ T cells that could be used to better understand responses to immunotherapy.
Identifying Therapeutic Targets and Evaluating Investigational Therapies
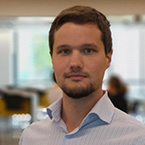
Other presentations from the session demonstrated how bioinformatics and artificial intelligence could be used to identify potential therapeutic targets and to predict the effects of small molecules. To identify potential targets of synthetic lethality, Miika Ahdesmaki, associate director of bioinformatics at AstraZeneca, utilized data from The Cancer Genome Atlas to map genetic alterations in specific cancer cell lines and examined data from genetic screens performed in these cell lines. This approach allowed Ahdesmaki and colleagues to identify genes and pathways that were essential in various cancer subtypes. For example, this strategy revealed that the CEBPB gene may be essential for the survival of CDKN2A-mutated lung squamous cell carcinoma cells, suggesting that CEBPB could be targeted in patients with this cancer subtype. Ahdesmaki and colleagues are currently investigating the therapeutic potential of several candidate targets.
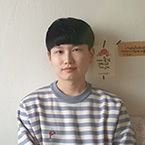
In another presentation, Junhyeok Jeon, a graduate student at Korea Advanced Institute of Science and Technology, described a deep learning model designed to predict the effects of small molecules on gene regulation. As explained by Jeon, deep learning is a form of machine learning that can identify important features from an input based on prior learning from a big dataset. The model developed by Jeon and colleagues, called DeepCGR (for Compound on Gene Regulation), utilized the structure of a small molecule compound and the sequence of the protein targeted by the compound to predict how the compound might affect the expression of various genes. Jeon and colleagues validated DeepCGR in multiple cancer cell lines representing different cancer types. This model could be used to better understand the impact of investigational therapies on cancer cells.
Resources for Researchers and Clinicians
Multiple presentations from the session described recently developed platforms designed to aid researchers and/or clinicians in various ways, including detecting genetic aberrations, searching and analyzing large datasets, and matching patients with relevant clinical trials.
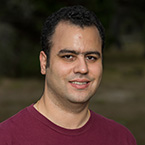
A presentation by Roozbeh Dehghannasin, PhD, a postdoctoral researcher at Stanford School of Medicine, described a statistical model designed to detect gene fusions at a single-cell level. Many gene fusions are known to drive cancer development, but limitations in current technologies have hindered fully understanding their functions. The model developed by Dehghannasin and colleagues, called SICILIAN (for SIngle Cell precIse spLice estImAtioN), can be integrated into conventional splice alignment tools to detect different RNA variants, such as splice junctions, gene fusions, and circular RNA. Dehghannasin and colleagues found that SICILIAN detected gene fusions with similar sensitivity but with greater specificity than an existing gene fusion detection tool called STAR-Fusion. SICILIAN represents a resource that could help researchers identify and understand gene fusions and their impact on cancer.
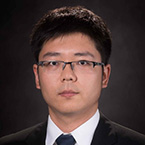
In another presentation, Jun Li, PhD, an assistant professor at The University of Texas MD Anderson Cancer Center, explained how a platform called DrOncoRight could assist researchers with searching and analyzing datasets. DrOncoRight allows users to type biological questions in plain language and receive answers from the system. The platform can guide users to the appropriate dataset for their question, and it can also perform data analyses and produce graphs. Furthermore, DrOncoRight has the capability to assess reproducibility of published scientific research.
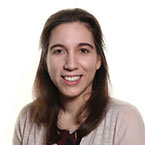
Finally, Tali Mazor, PhD, a computational biology scientist at Dana-Farber Cancer Institute, reported on MatchMiner, an open-source platform designed to match patients with relevant clinical trials by mining information about the patient and their cancer, as well as eligibility criteria of various clinical trials. As Mazor explained, the resource could be used to help individual patients receive appropriate treatment and to recruit eligible patients into clinical trials.
Together, the presentations from this session highlight the multitude of ways that bioinformatics and artificial intelligence can be used to drive cancer research and improve patient care. As noted by Dry, “Now, more than ever, computational techniques are at the forefront of our ability to drive discoveries and ideas…to help cancer patients.”